featured insight
featured insight
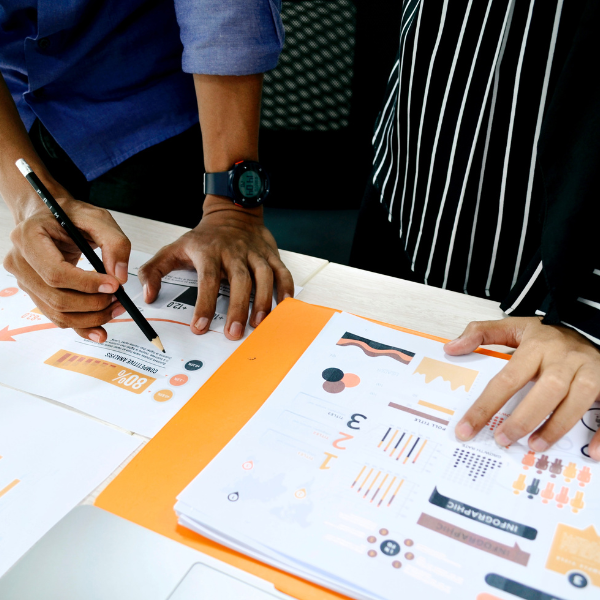
Margin of Error in Survey Research
Margin of error is a very specific measure of sample bias, and it can help you determine how accurate a survey is likely to be. But it doesn’t account for every bias that might impact the accuracy of a survey.
To get to more accurate results, survey researchers must contend with bias, both their own and their respondents’.
Within the context of survey research, bias describes behaviors or actions by either the researcher or respondents that cause the outcome of the survey to deviate from the true value or information.
If you are particularly eagle-eyed, you might have noticed a “margin of error” number when looking at a public opinion research. Margin of error is a very specific measure of sample bias, and it can help you determine how accurate a survey is likely to be. But it doesn’t account for every bias that might impact the accuracy of a survey.
This post will explain what the margin of error covers and how it is calculated. We’ll also review a few other types of bias to help you evaluate how accurate a survey is likely to be.
What Is Margin of Error?
The point of survey research is to learn more about the opinions of a group of people without having to ask every single individual in that group. So surveys use samples: a selection of people in a group rather than the entire group. The polling industry commonly uses the analogy of going to the doctor to get your blood tested. In the same way your doctor draws just a sample of blood to send to the lab for testing, survey researchers take a sample of the public to poll.
Done correctly, the sample will be representative of the universe we’re polling, and so it will accurately measure public opinion. The margin of error is a measure of how far we would expect a representative sample to deviate from the true value.
To dive deeper into how this is calculated, the margin of error is based on three factors: the confidence level (more on that in a moment), the number of people in the total population being researched, and the sample size of the survey. Let’s break each of these down:
Confidence level: This is the probability that the results of the research will be accurate—that they will capture the correct opinions of the population being surveyed. Confidence level set before the research takes place and helps determine the sample size. Most of the time, researchers use 95% as their confidence level.
Total population: This is the total number of people in the group being researched. For example, a company might want to know the opinions of all their current customers; so the total population would be the number of their current customers. In political survey research, researchers often use registered voters, which at the time of the last election numbered about 186 million.
Sample size: This is the number of people surveyed.
How Confidence Level and Sample Size Impact Margin of Error
The smaller the sample size when compared to the total target population, the higher the margin of error. For example, if a researcher polls 1,000 people and the target audience is registered voters in the United States, the margin of error is ±3.1%. If a researcher polls 100 people in that same target audience, the margin of error jumps to ±10%. The only way to lower the margin of error is to have a larger sample size. When determining the appropriate sample size, the sampling bias must be balanced against the timeline and the budget.
Confidence level also impacts the margin of error. The higher the confidence level, the larger the margin of error. That’s because the more certain you want to be (while keeping sample size the same), the more likely it is that the survey research will miss the mark.
Margin of Error Example
Now that you’ve got the basics, let’s look at an example. Let’s say that an organization wants to know the favorite pizza topping of American adults over the age of 18. The organization decides they want a confidence level of 95% (as is the standard in public opinion research), and the researcher suggests a sample size of 1,000 people.
When the research comes back, the results show that 75% of people think pepperoni is the best pizza topping. Based on the confidence level (95%), the size of the total target population (200 million people), and the sample size (1,000 people), the survey has a ±3% margin of error.
Now let’s put that margin of error to work. First, add the margin of error percentage to the result (75%) to get 78%. Then, subtract the margin of error from the result to get 72%. Since the confidence level is 95%, we know that there is a 95% chance that between 72% and 78% of American adults think pepperoni is the best pizza topping.
Types of Bias that Margin of Error Doesn’t Account For
It’s important to note that the margin of error only concerns itself with the sampling bias. There are other types of bias that survey researchers must contend with to get the most accurate results possible. Let’s look at three of those: nonresponse bias, answer order bias, and question wording bias.
Nonresponse Bias
Nonresponse bias happens when the people who answer a survey are different in some systemic way from people who don’t, which biases the results. For a survey to be accurate, the sample must reflect the total target population, not just part of it. Maybe those who answer the survey are younger than those who don’t, or they’re wealthier, or they’re more engaged. Any of these factors can skew results.
Let’s look at an example. You’ve probably seen that picture of a smiling Harry Truman holding up a Chicago Daily Tribune newspaper with a headline proclaiming “Dewey Defeats Truman.” This was the expected result heading into the 1948 election, as polling—still in its infancy as an industry—had widely expected Dewey to prevail. But, the polling was conducted over telephones, and in 1948 there were still a lot of people who didn’t have telephones. The households without telephones were more likely to vote Democratic than Republican, so the polling ended up oversampling Republicans and overestimating Governor Dewey’s support.
Answer Order Bias
In multiple choice questionnaires, survey respondents are more likely to pick the first answer or the last. There are many reasons for this: some respondents are rushing, others pick the first option they agree with (even if there is a better option later), and others find the last option the most memorable when they go to answer. So, in survey research, sequence matters.
When good survey researchers test a series of messages, they rotate the order of the messages to minimize the answer order bias. In political survey research, the names of candidates should always be presented in a rotating order. Rotation creates a level playing field between the topics and reduces any undue weight given to one answer over another.
Question Wording Bias
Question wording bias occurs when a survey question is written in such a way that it encourages or discourages a specific answer, leading to inaccurate results. Some obvious examples come from the political sphere (“Do you stand with candidate X or do you want our country fall into economic decline?”)
But even question wording differences that seem small can result in skewed data. This bias is often so subtle that people administer the same survey for years, getting erroneous results that reinforce themselves over and over.
For example, a survey question that asks, “Are you in favor of a new traffic light at the intersection of Johnson Street and Maple Street?” is likely going to get different responses than a question that says “Are you in favor of traffic safety measures at the corner of Johnson Street and Maple Street?” The way that survey researchers choose to phrase questions can subtly direct readers to certain answers, which is why creating an unbiased questionnaire is one of the most important steps in the research process.
While it is impossible to completely eliminate every potential source of error in survey research, the best researchers will employ a variety of strategies to mitigate the primary types of bias to achieve the most accurate results possible. If you’re looking for accurate, actionable data to plan the next steps for your organization, business, or campaign, we can help. Get in touch or subscribe to our newsletter.
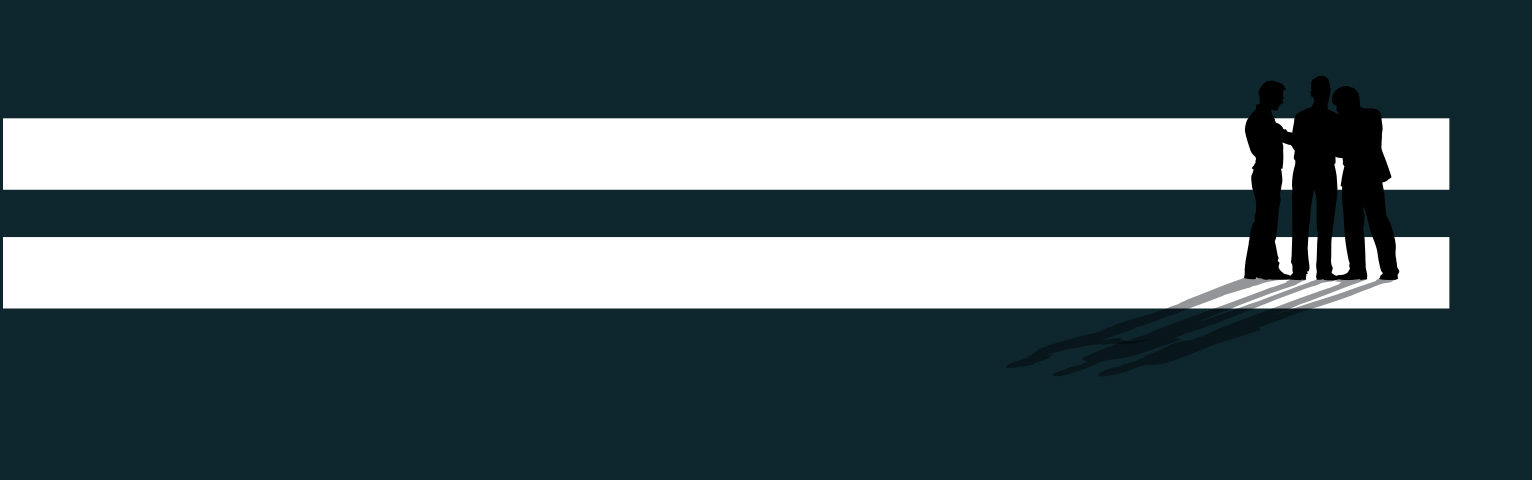